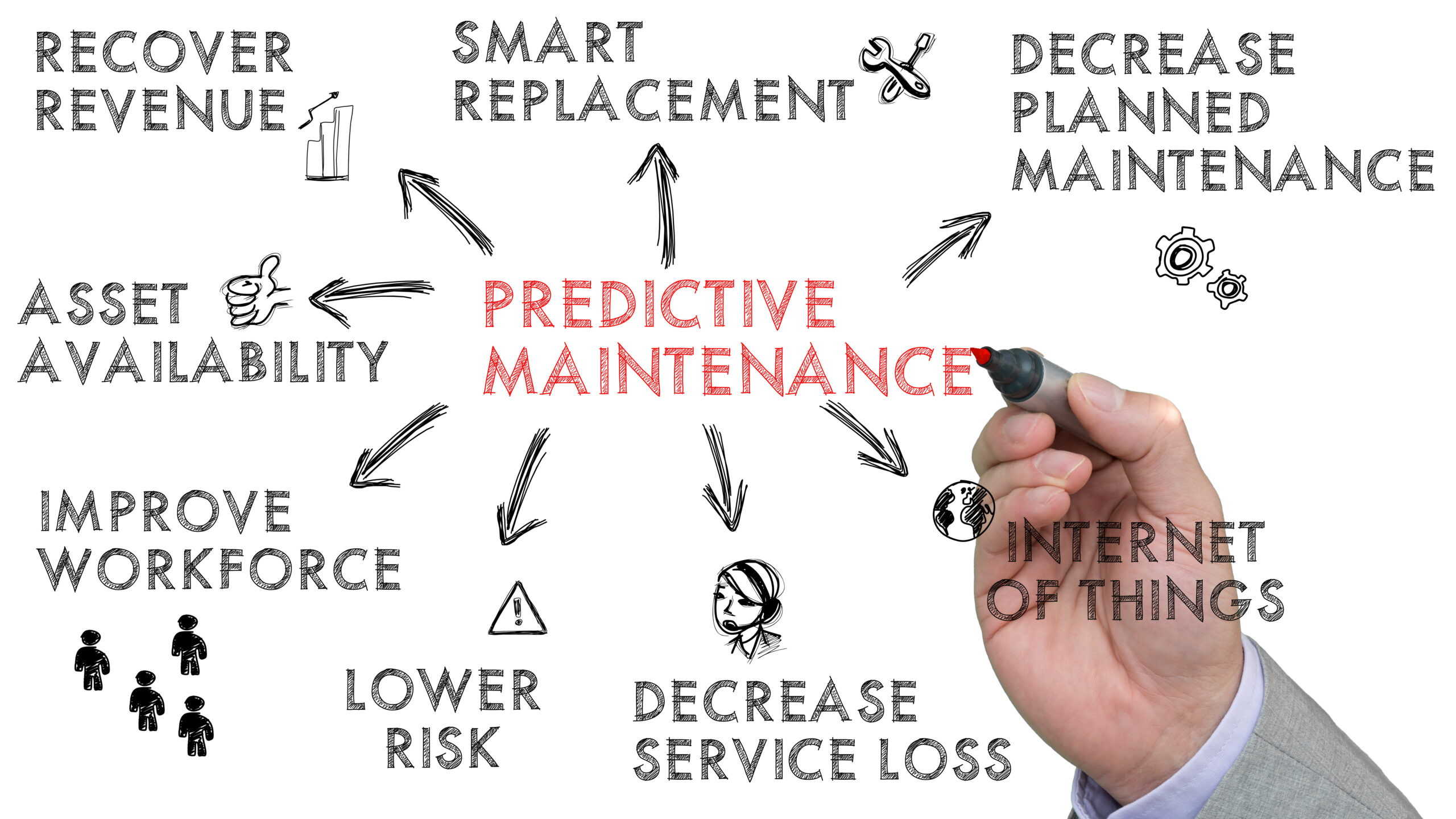
Managers and engineers are no fans of downtime, to say the least. If they had one wish, they would choose to keep their assets in working condition 24/7, every single day of the year. The way maintenance is done has to be changed to get closer to this goal.
Classic maintenance scheduling is based on the suggested maintenance routines and on-floor experiences. This keeps machines and electronics systems running but remains inefficient and increases risks of wear, breakages, and downtime since it doesn’t use real-time data. Unplanned downtime alone is estimated to cost industrial manufacturers about $50 billion a year. With the adoption of Industry 4.0 tech, industries can achieve near-zero downtime using predictive maintenance.
What is Predictive Maintenance?
Predictive maintenance has been around since the 90s but it has come a long way since then. The aim of predictive maintenance is to schedule maintenance based on the real-time data collected using embedded sensors from across the factory. The data used by such systems may include temperature, power consumption, the performance of subsystems, and other important metrics that help ascertain the health of plant operations.
This data has huge importance in the fourth industrial revolution. Cloud computing and big data analytics can generate valuable information about equipment performance, including valuable information about predictive maintenance. A maintenance system equipped with AI and ML can use the data to predict which parts of the system may require maintenance soon.
Predictive maintenance can improve productivity, reduce downtime, and save assets from failure, all of which directly help the bottom line. Here are six steps you can follow to set up an efficient predictive maintenance plan for your factory.
Analyze the Needs and Inventory the Assets
The first step towards a predictive maintenance plan is doing an inventory of the assets and assessing the system. Analyzing processes brings the need for predictive maintenance, and inventory makes it easy to find assets that require predictive maintenance the most.
Choosing assets for a pilot predictive maintenance plan is simple – the assets that affect operations significantly have high repair/replacement costs and ones that require expensive specialist attention, which may take a while. This will help get the highest return on investment by cutting maintenance costs of the most critical assets.
Collect Equipment Data
Once you know which assets will be part of the predictive maintenance plan, you need all the available data about them. The data helps in creating a primary predictive maintenance model. The CMMS then uses the model to predict maintenance requirements. Using such data also saves time and gives a head start to the model which can learn from the history of the assets.
Modern systems usually have a large amount of data about assets and their performance history. Apart from the digital data, you can collect historical manually recorded data, information from manufacturers, and experience-based information from workers.
The data you collect at this stage also provides more information on which assets deserve predictive maintenance. If a particular asset’s maintenance costs were not accurate before, now you’ll get a better idea. For example, if a component in your system causes breakdowns at many locations, it should be on the predictive maintenance plan.
Conduct Failure Analysis
After you have enough data about the assets, you can run a failure analysis to identify different modes of failure – how an asset can break down. The analysis includes finding out the extent, effect, frequency, and difficulty in the identification of failure. Failure Mode and Effect Analysis (FMEA) is a popular and effective method to achieve these goals.
FMEA is a step-by-step process of identifying different failure modes and their effects on any product to assign priorities. FMEA is a complex process and takes into account the severity, frequency, and detection variables as discussed. The final result is the prioritized list which you can use to create the prediction models for high-risk assets first. After completion, the primary model is prepared for the CMMS to implement.
Modern CMMS platforms can also provide the data visibility needed to identify which issues are causing the most disruption and therefore costing the company the most. These can then be prioritized, and a good system will enable you to manage the resolution of issues at their root cause.
Upgrade Data Collection Sensors and Equipment
At the center of any predictive maintenance plan is the data collected by sensors. If the data is inaccurate or incomplete, even the best theoretical model might become inefficient. To ensure accurate and complete data collection, you may need extra sensors or equipment.
Your existing CMMS might have sensors and equipment which can be upgraded to meet the requirements. Different sensors like current and voltage sensors, thermometers, and cameras are common in electronics systems. The CMMS uses the real-time data from these sensors to function effectively and predict maintenance requirements.
Algorithm Development with AI and ML
Like data, algorithms are also central to predictive maintenance. Algorithms utilize the collected data to provide the alerts you need. Predictive maintenance uses system monitoring and predictive algorithms as part of data analysis.
In system monitoring, CMMS uses real-time data from sensors to point out any changes that may be due to asset wear. This is done using multiple variables and previously recorded parameters for failure conditions. Predictive algorithms provide the estimated remaining time to failure for the asset based on a comparison of its recent and historic data. These algorithms may be created using modeling with artificial intelligence and machine learning.
The predictive modeling is prepared by an expert data scientist. The model is then combined with a machine-learning platform that makes changes to the algorithm with each failure. The results provide improved predicting capabilities and near-zero unplanned downtimes.
Deployment
Now that the sensors and predictive algorithms are ready, you can integrate the technology with chosen assets. When beginning with limited assets, you can run the algorithms on embedded devices mounted near the asset since the amount of data isn’t large. As you integrate more assets with the plan, you might have to move towards cloud computing to collect and analyze data at faster speeds. Once the plan is deployed, CMMS will provide notifications for maintenance predictions.
An efficient predictive maintenance plan is a necessity for modern systems in any industry. While setting up a predictive maintenance plan is a complex task, it is typically worth the returns. According to a McKinsey report, predictive maintenance can help bring down maintenance costs by 10 to 40 percent and cut downtime by 50 percent. The steps listed here can simplify the task of laying down a predictive maintenance plan with help of skilled engineers.
About the Author
Eric Whitley has 30 years of experience in manufacturing, holding positions such as Total Productive Maintenance Champion for Autoliv ASP, an automotive safety system supplier that specializes in airbags and restraint systems. He is also an expert in lean and smart manufacturing practices and technologies. Over the years, Eric has worked with all sectors of industry, including Food, Timber, Construction, Chemical, and Automotive, to name a few. Currently, he’s a part of the L2L team.