Quality has been a well-defined term in manufacturing for decades. Quality is when the product created meets the original expectations for product performance and features. Traditionally, quality control has been delegated to specialists and often relegated to specific, isolated steps in the overall manufacturing flow. All that is changing due to the adoption of digitalization, which has led to a paradigm shift for the emergence of an innovative approach called closed-loop quality (CLQ).
Due to digitalization, today’s smart manufacturing can now provide crucial, real-time, quality data that enables manufacturers to continually adjust their processes to optimize quality in real time. When real-time process adjustments are impossible, CLQ identifies parameter trends and their sources to allow adjustment at the first available downtime. With the help of CLQ, when a product’s quality or the manufacturing process becomes subpar, the manufacturer can quickly identify and correct nonconformance when practical. This leads to overall improved product quality while minimizing scrap and rework.
With CLQ, quality-related concerns become a shared responsibility instead of a disconnected or isolated process occurring at the end. The CLQ process involves everyone from the design and engineering process to the manufacturing personnel and quality team.
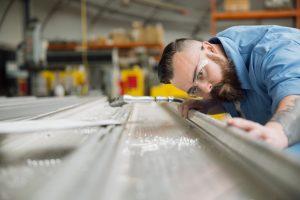
Pursuing a quality culture
CLQ provides complete visibility of quality-related metrics from planning through delivery of the completed product. It also can improve the overall product quality over time, but where do manufacturers start? There are the key stages to implementing CLQ:
- First, it is crucial to start in the machine design stage with a quality mindset by listening to the voice of the customer to detail all the requirements, including the one for quality, and carefully defining all the capabilities and expectations of the product in a virtual environment before building the first prototype. This includes detailing quality procedures and identifying best practices to follow from the standards.
- Then, it is fundamental to write a plan for quality upfront and use the recommendation of standards to apply risk analysis for expected failure modes or creating procedures for mitigating those issues. These requirements could be as design specific as defining vibrations, temperature tolerances, or process definition oriented to set machine conditions, even looking at environmental and machinery conditions that may impact quality and the overall production processes. The plan also should describe how and where to monitor these requirements to guide the final product to meet the predefined quality goals.
- An essential stage is to implement ongoing quality checks throughout the production process that monitor the specifications described in the quality plan. This involves capturing the output parameters and comparing the as-built conditions against the requirements. It enables the manufacturing team to quickly resolve any disturbing parameter trends or deviations from the quality plan.
- Nevertheless, it is crucial to continuously improve the overall quality level, not only in case of a major issue, but also to pursue a real path to quality excellence. The continuous monitoring and assessment of quality core processes and improvement projects will lead to a continuous cooperation across departments to constantly deliver great products.
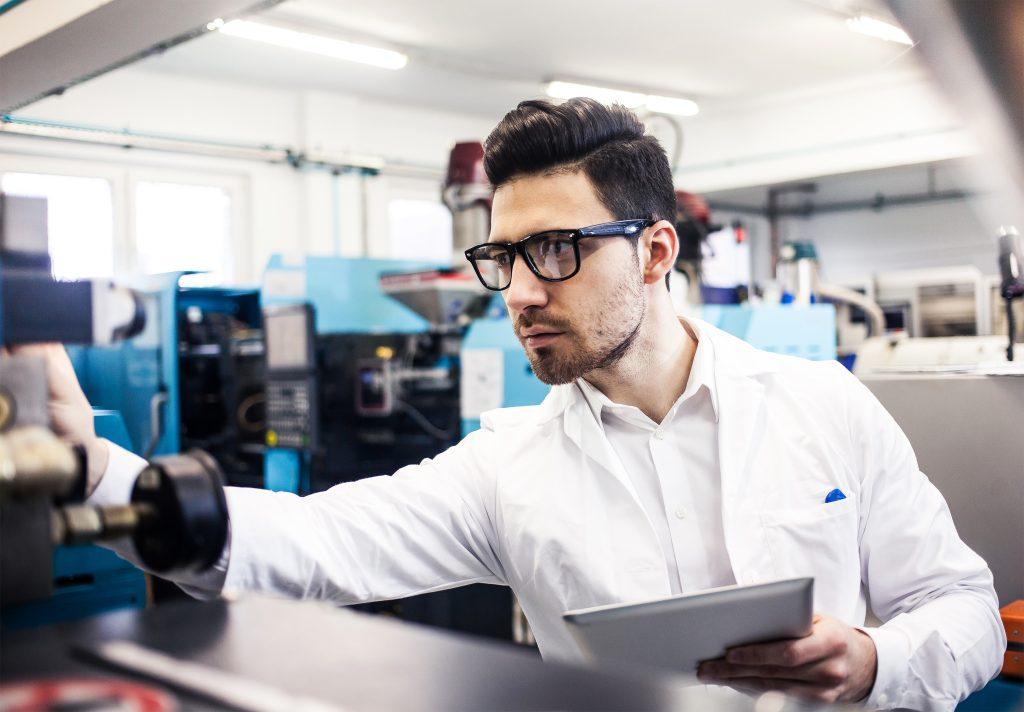
It is all about the culture and data
A shift in the quality culture is the foundation of a new paradigm. And quality analysis can be increasingly consistent by using reliable data. Quality information is collected and shared in repository with the engineering domain by leveraging a common collaborative platform. In the production environment, to ensure sufficient data is captured, most manufacturers must augment older machines and key areas in their facilities with IoT devices. These devices need to be carefully positioned to supply the uninterrupted and unhindered data collection necessary to transfer closed-loop feedback to the manufacturing process. Alternatively, where possible, manufacturers can leverage smart machines – cognitive computing systems – to seamlessly collect and deliver this invaluable data.
To facilitate the collection and analysis of this data, information technology (IT) is converging with manufacturing operational technology (OT) to analyze large, complex data sets, making it possible to look at the OT world holistically rather than just at the individual, siloed units of a single machine or production line.
With IT/OT convergence, new patterns emerge, and new insights are revealed, allowing data-driven decisions in near real-time. This in turn makes it possible to apply machine learning and data analytics to the operations, increasing manufacturing adaptability and making the manufacturing process easier to scale.
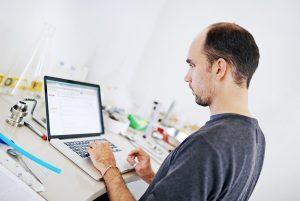
Ongoing benefit: Continuous quality improvements
With a quality plan in place and sufficient data collection across the factory, manufacturers can verify the product quality produced against the engineering requirements throughout the development and manufacturing process. This scenario creates an environment of continual optimization of critical quality characteristics throughout the product’s lifetime. It is possible to broaden this closed-loop quality process to supplier quality management to ensure the availability of high-quality materials even when experiencing unforeseen supply chain disruptions.
In my discussions with customers, companies implementing closed-loop quality processes typically see a median product compliance rate of 99 percent, with most performing in the 97 to 100 percent range. Organizations that have not implemented closed-loop quality experience greater variations in quality, with most performing in the 90 to 98 percent range. These improvements in quality outcomes might seem small, but they can provide a significant competitive advantage. It can be the difference between being first or second to market, avoiding a costly product recall, or giving a manufacturer the crucial edge for winning future contracts.
Smart machines ideal for closed-loop quality
While in many brownfield facilities IoT devices are used to supply uninterrupted data collection and IT/OT convergence to analyze complex data sets comprehensively, in new factories or on new production lines, smart machines, or cognitive computing systems, they can also be implemented to provide ideal quality on the factory floor.
Today’s smart machines create more value for the manufacturing customer by supplying better quality data and higher reliability—all suited to supporting a CLQ approach. Other benefits of using smart, networked machines are lower costs, improved safety, less waste, and better serviceability. Smart machines inherently improve overall quality by preemptively detecting problems, enabling the manufacturer to respond in real time before any nonconformance becomes a major issue. This helps minimize significant downtimes, boosting the overall factory productivity without compromising optimal quality.
A notable example of using smart machines to create value in a CLQ process is a customer in Germany that collects and analyzes machine data and provides users with recommendations on how to optimize their smart machine operations. The company relies on lasers to continuously measure the tooth width of the gear production machine, evaluate the measurements in real time and adjust if necessary. It is exact in delivering the correct product and, if needed, stopping the production process if it detects a potential issue with product quality. This process has helped reduce maintenance costs by 10 to 40 percent and energy consumption by 40 percent. The result is a highly agile and adaptable production environment for creating customized, personalized goods.
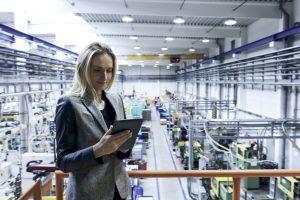
Developing a manufacturing digital twin
With CLQ in place, a digital twin of the manufacturing environment can be created and enriched with real-time data. The digital twin is a virtual, digital replica of the entire manufacturing environment that combines the virtual and physical worlds to provide new insights and efficiencies for product manufacturing through data analytics and AI. With a comprehensive digital twin in place, the virtual representation can be used to analyze any threats to quality, providing a crucial feedback loop between manufacturing operations and quality.
In addition, it provides manufacturers with a mechanism to understand what could happen in the future. They can confidently use the digital twin for exploring scenarios for optimizing operations, predicting maintenance, enabling more efficient commissioning, better changeovers, and faster production line changes. All this encourages managers to examine a broader range of feasible approaches for quality enhancements before committing to physical implementation on the production floor.
Looking to the future
With a robust CLQ approach and a digital twin of the production environment, manufacturers can evaluate and adopt more advanced technological capabilities. For example, they can efficiently determine how to deploy robots and cobots for optimal interaction with skilled workers.
As reshoring and localization for production become more pervasive, hyper-automation—that relies on highly flexible machines to provide next-generation performance—can be intelligently applied where it will have the most impact. It is even possible to have fully integrated product lifecycle management (PLM) systems with a native quality management system (QMS) extending down the supply chain to suppliers and customers to ensure customer requirements to the design and the production shop floor are connected and working effectively.
By taking the above-mentioned steps, manufacturers can improve product and manufacturing quality and performance continuously to realize the immediate benefits of CLQ, today. And in the long term, this approach opens a host of possibilities that will have a tangible impact on the manufacturing bottom line and, ultimately, customer satisfaction.
By: Rahul Garg, Vice President for Industrial Machinery & SMB Program, Siemens Digital Industries Software